In today’s rapidly evolving digital landscape, data underpins virtually every aspect of business operations, from strategic decision-making, to regulatory compliance and customer engagement. As the volumes and complexity continue to surge exponentially, compiling and maintaining high-quality data has become increasingly important. Simply accumulating vast datasets is not enough; the true value of data lies in its quality.
The consequences of poor data quality are staggering. According to Gartner, businesses lose an estimated $12.9 million annually due to insufficient data, affecting everything from operational efficiency to customer satisfaction and regulatory compliance. Another study by IBM puts the cost of poor data quality at $3.1 trillion per year for the U.S. economy alone, which highlights just how widespread and damaging the issue is. It’s not hard to see why. Poor data leads to poor decisions, flawed analytics, ineffective marketing, compliance violations, and reputational damage.
The data explosion and its challenges
Data volumes are expanding at an unprecedented rate. In 2023 alone, approximately 328.77 million terabytes of new data were created daily, and this figure is projected to increase exponentially in the coming years. The challenge is not only managing such vast quantities of data but also ensuring its integrity.
Businesses once relied on structured, manageable datasets. Now, they must deal with unstructured and semi-structured data from sources like social media, IoT sensors, multimedia content, and real-time transactions. This shift has made data quality management far more complex, requiring new approaches such as data observability tools, automated validation, and AI-driven quality assurance.
Data quality and Business Intelligence
Business intelligence (BI) is only as good as the data behind it. BI tools help organizations analyze trends, forecast demand, and optimize resources, but if they rely on inaccurate or inconsistent data, the results can be misleading at best or disastrous at worst.
Consider a financial services firm using flawed credit risk assessments. It might accidentally approve risky loans while rejecting creditworthy applicants, leading to unnecessary losses and customer frustration. In retail, inaccurate inventory data could cause stock shortages or overstocking, disrupting supply chains and eating into profits.
Poor data quality is an even more significant risk for companies investing in AI and machine learning. These technologies depend on high-quality training data to make reliable predictions. The outcomes can be dangerously misleading if the data contains errors, biases, or inconsistencies. In healthcare, for instance, incorrect patient records or flawed medical imaging data could lead to misdiagnosis, putting lives at risk.
Customer experience: the hidden cost of poor data
Today’s consumers expect personalized interactions, seamless digital experiences, and accurate information. When businesses rely on outdated or incomplete data, the result is frustration, lost trust, and lost revenue.
Take marketing personalization gone wrong. If customers receive promotions for items they’ve already bought, irrelevant recommendations, or duplicate emails, their confidence in the brand erodes. Shipping errors or incorrect billing information, often caused by flawed customer records, can turn an otherwise smooth transaction into an exercise in frustration.
Conversely, companies investing in data quality directly benefit customer engagement and retention. Netflix and Spotify are prime examples. Using clean, structured, and valid data, they deliver hyper-personalized recommendations that keep their users engaged. Netflix estimates its recommendation system alone saves the company over $1 billion annually by helping customers find content they’ll enjoy—something only possible with the highest-quality data.
Compliance, risk management and regulations
Data quality isn’t just about business efficiency; it’s also a regulatory necessity. Frameworks like the Basel Committee on Banking Supervision’s standard number 239 (BCBS 239 ) impose strict data accuracy, traceability, and security requirements. Given how poor data quality can lead to inadequate risk assessment, amongst other issues, the BCBS 239 outlines principles for effective risk data aggregation and reporting. Financial institutions failing to adhere to these principles risk regulatory scrutiny, financial penalties, and significant reputational damage.
Under the General Data Protection Regulation, personal data must be “accurate and, where necessary, kept up to date.” This means businesses must actively monitor and correct errors in their customer records to avoid penalties. Financial institutions face even stricter requirements, needing precise and verifiable data to prevent fraud, money laundering, and economic crimes.
Beyond regulatory risks, poor data quality can expose businesses to cybersecurity threats. Outdated access control lists can leave unauthorized users with system privileges, while inconsistencies in security logs can make it harder to detect threats. As data security concerns grow, ensuring data quality is increasingly essential to risk management.
Achieving and maintaining high-quality data
Understanding the importance of data quality is one thing; implementing a strategy to maintain it is another. Organizations need a multi-layered approach that combines technology, governance, and cultural shifts to ensure data remains accurate, complete, and usable.
- Automating Data Quality Processes: Manual data cleansing is slow and prone to errors. Businesses should use automated tools for data profiling, anomaly detection, and real-time validation to catch and fix issues proactively.
- Implementing Data Governance Frameworks: Clear policies on data ownership, standardization, and accountability help ensure consistency across departments and prevent data silos.
- Adopting Data Observability Practices: Real-time monitoring tools can detect and correct anomalies before they impact decision-making. Platforms that track data health, lineage, and usage provide visibility into data pipelines.
- Fostering a Data-Driven Culture: Data quality isn’t just an IT problem, it’s a company-wide responsibility. Training employees on best practices and encouraging accountability improves overall data integrity.
The future of data quality
As data grows in volume and complexity, its quality will become an increasingly more significant factor in determining business success. Today, organizations that invest in data quality gain a competitive edge in analytics, customer engagement, and regulatory compliance.
The real question isn’t whether data quality matters; it does. The question is whether companies are ready to face the challenges of an increasingly data-driven world. Those who prioritize high-quality data will thrive. Those who ignore it will find themselves drowning in a sea of unreliable information.
References:
- Gartner – Report on annual losses due to poor data quality (~$12.9 million per organization)
- IBM – Study estimating bad data costs the U.S. economy $3.1 trillion annually
- Dataversity – Discussion on data quality and business impact
- Netflix Tech Blog – Data-driven recommendation system saving $1 billion annually
- General Data Protection Regulation (GDPR) – Compliance requirements for data accuracy and governance
- California Consumer Privacy Act (CCPA) – Data protection and regulatory considerations
- BCBS 239 Compliance: A Guide to Effective Risk Data Aggregation and Reporting
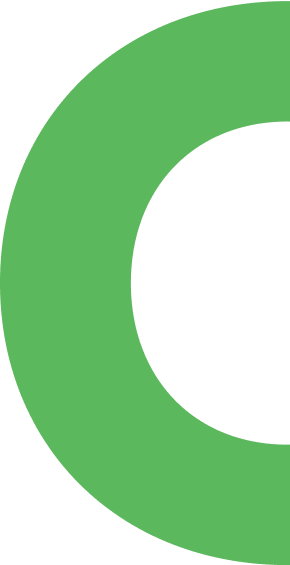
Why is the importance of Data Quality growing
In today's rapidly evolving digital landscape, data underpins virtually every aspect of business operations, from strategic decision-making, to regulatory compliance and customer engagement. As the volumes and complexity continue to surge exponentially, compiling and maintaining [...]
Case study: SEB Baltics
Improving data quality and compliance Case study with SEB Baltics THE CUSTOMER SEB Baltics provides universal banking including advisory services to private individuals and all corporate customer segments in Estonia, Latvia and [...]
Release 2025.2
Starter edition of the platform A free version of SelectZero platform for data quality management is now available, making it easier than ever to improve data reliability. More information about the functionality is available here [...]
Case study: Citadele Bank
Ensuring data quality & regulatory compliance in banking Case study with Citadele THE CUSTOMER Citadele is a universal bank offering a wide range of banking products to retail, SME, and corporate customer [...]
Release 2025.1
Sharepoint connection for Excel files You can now connect to SharePoint to use Excel files as a data source, making it easier to track and validate dynamic data. This ensures real-time issue detection [...]
Release 2024.12
Advanced data lineage You can now view column-level lineage directly within the object lineage chart, enabling detailed tracing for individual columns. Additionally, we’ve made it easier to share and analyze lineage data with the [...]