Data is an integral part of all our business processes. The activities carried out in a business process need data, and the activities produce data. So, we cannot look at business processes without data, and vice versa. Here are some business processes in which high quality data plays a vital role.
Identifying and managing risks more effectively
High quality data is crucial in business processes for identifying and managing risks in the financial sector. It can help address significant market volatility that affects loan paybacks or investment portfolios. Data on a client’s credit history, employment status, and repayment of previous loans can assist in assessing the creditworthiness of potential borrowers, enabling the identification of high-credit-risk customers who may face difficulties in repaying their loans.
Similarly, data on market conditions, historical security prices, interest rates, and economic indicators can be used to predict potential market movements and their impact on the investment portfolio. This predictive analysis can help reduce potential losses and result in a more stable and resilient investment portfolio.
Regulatory and financial reporting are of utmost importance in this context. Accurate and timely regulatory reporting ensures that a company meets all legal and statutory requirements, which is crucial for maintaining operational legitimacy and avoiding penalties. It also fosters transparency and trust with stakeholders, including investors, regulators, and customers. Financial reporting provides a clear and comprehensive picture of the company’s financial health, aiding in strategic decision-making and fostering investor confidence. Robust financial reporting practices help track financial performance, identify trends, and make informed predictions, thus supporting overall business stability and growth.
Additionally, data on regulatory updates, compliance audit results, and transaction data can assist in identifying compliance breaches or suspicious activities. This can decrease the likelihood of fines and improve a company’s reputation.
Decision-making
Gathering high-quality data during the company’s various business processes is essential for better management decisions. Data collected from production processes, including machine performance, line efficiency, defect rates, and maintenance schedules, can help make better decisions about machine maintenance needs and line optimization.
Similarly, data collected from supply chain processes, such as supplier performance, stock levels, waiting times, and transport logistics, can assist in better decision-making regarding stock levels and supplier selection. Furthermore, data on customer service levels, feedback, product return rates, and customer satisfaction rates can improve customer service strategy decisions.
Ensuring customer satisfaction and improving loyalty
Accurate data in customer satisfaction and loyalty business processes can help address issues related to customer retention, inadequate customer service, and understanding customer needs. Data from customer feedback forms, surveys, and social media (comments or mentions of a product/service) can help identify the reasons behind customer dissatisfaction. This, in turn, can help determine which aspects of the product/service offering need improvement to enhance customer satisfaction.
Understanding and complying with the General Data Protection Regulation (GDPR) in these efforts is crucial. GDPR requires the protection of customer data and promotes transparency and trust between businesses and their customers. Customers’ confidence in the company increases when they are assured that their personal information is handled carefully and used ethically. This trust can significantly enhance customer satisfaction and loyalty, as customers feel more secure and valued. Ensuring GDPR compliance can thus serve as a competitive advantage, fostering stronger relationships and long-term loyalty.
Data on customer touch points (such as website clicks or email activity) and e-commerce activity can help suggest products that interest customers, ultimately boosting customer engagement and conversion rates. Additionally, data on participation in loyalty programs, reward redemption, and customer spending patterns can provide insights into how loyalty programs impact customer purchases and returns. This information can be used to design more effective and engaging loyalty programs.
Improving inventory management, high operating costs, and late delivery times
Effective data management in logistics and supply chains can address common issues such as delayed deliveries, high operational expenses, and warehouse management challenges. By leveraging real-time data analytics and automation, companies can ensure timely deliveries and reduce operational costs significantly. Additionally, streamlined data management enables better inventory tracking and warehouse optimization, leading to improved overall efficiency in the supply chain.
Data regarding product sales trends, seasonal consumption fluctuations, and warehouse turnover can assist a company in forecasting product demand, ultimately leading to better inventory management. By analyzing sales trends, businesses can predict which products will be in high demand and when, allowing them to stock appropriately. Understanding seasonal consumption patterns helps companies adjust their inventory levels to match peak and off-peak periods, reducing the risk of overstocking or stockouts. Additionally, monitoring warehouse turnover rates enables more efficient use of storage space and ensures that inventory is managed in a cost-effective manner.
Information on transportation routes, duration, and costs can enable companies to plan the most efficient delivery routes, resulting in faster deliveries, improved reliability, and reduced fuel expenses. By analyzing route data, companies can identify the shortest and least congested paths, ensuring quicker transit times for deliveries. Accurate cost assessments allow businesses to choose cost-effective routes, ultimately lowering fuel expenses and operational costs. Moreover, reliable planning leads to consistent delivery schedules, enhancing customer satisfaction and trust in the company’s services.
Data related to product deliveries, customer feedback, and return rates can help identify patterns and causes of late deliveries and returns. By analyzing these patterns, companies can implement targeted improvements in product quality assurance and optimize delivery processes to enhance overall customer satisfaction.
Not just data, but high quality data!
Did you notice that references were made not just to data but to high quality data in several places above? You’ve probably heard the expression “Garbage-In-Garbage-Out” when people talk about data quality. One of the most important criteria is that data must be high quality. Otherwise, it cannot be used in the business processes and decisions mentioned above because it is simply too inaccurate, insufficient, unreliable, etc.
But what are the characteristics of high-quality data?
- Completeness — Data must be complete. Data is complete when no records or essential information for that particular object are missing from the database. For example, if a person’s health record is missing information about allergies or medication used, then the person’s health record is not complete.
- Consistency — Data should be consistent. Data consistency refers to the consistency of data values (e.g., standard classifications have been agreed upon) and the correspondence across different datasets/systems over time. It means there are no inconsistencies or discrepancies in the data, ensuring the information remains reliable and accurate across various applications and reports.
- Timeliness – Data should be up-to-date and available when needed. Timely data means the information is up-to-date and available at the right time, ensuring that decisions are based on the most up-to-date information. Outdated data can lead to missed opportunities and decisions based on outdated information.
- Accuracy—Data should conform to defined formats, structures, and business rules. Accurate data meets all defined criteria and constraints, ensuring that it is fit for purpose and reliable for its intended purpose. Inaccurate data can lead to errors in data processing and analysis.
- Uniqueness—Data should not contain duplicates. Unique data ensures no redundancies or inconsistencies and prevents the accurate identification and management of units. Duplicates can distort analysis and reporting, leading to inaccurate decisions and conclusions.
- Integrity—Data should correctly maintain links and connections between entities. Data integrity refers to data’s accuracy, consistency, and reliability throughout its lifecycle. It ensures that data is not altered or corrupted during storage, retrieval, processing, or transmission and remains accurate and reliable.
- Reliability – Data should come from trusted and verified sources. Reliable data consistently produce the same results under the same conditions, ensuring they can be relied upon for decision-making and analysis. Unreliable data can lead to poor decision-making and loss of confidence in data-driven processes.
- Relevance—Data should be relevant and applicable in the context in which it is used. Relevant data directly supports the goals and decisions, ensuring the knowledge generated is meaningful and actionable. Inappropriate data can confuse analysis and obscure essential insights.
- Accessibility—Data should be easily accessible to those who need it. Accessible data shall be stored in a way that allows for their efficient retrieval and use, ensuring they are only accessible to those with the right to access them.
- Traceability—Data traceability means the ability to trace data history, use, and location. It includes the ability to trace the origin of data, track it through its lifecycle, and understand how it has been used, modified, and accessed. Traceable data helps maintain accountability, quality control, and regulatory compliance.
- Understandability—Data should be accessible for users to interpret and understand. Comprehensible data is precise, well organized, and presented in a way that allows it to be easily interpreted and used for decision-making. This includes using appropriate labels, measurement units, and formats that are familiar and meaningful to the target audience. Clear definitions, metadata, and documentation that give context and meaning to the data play an essential role in ensuring their comprehensibility.
How is high quality data created?
Generating high-quality data relies on well-designed data collection and processing business processes. These are supported by information systems or structured data collection templates. Before collecting data, it is important to determine where and from whom to collect it.
In information systems or templates that support data collection, data must be collected in predetermined forms, following specific rules. These systems and templates define how the data can be entered and ensure that it adheres to the predefined business rules.
Clear understanding of the work processes for collecting and processing data is essential for producing high-quality data. If data is collected with high quality at the data collection stage, less work is required at the data processing stage. Conversely, if data collection does not prioritize data quality, it leads to more work in the data processing phase. It’s important to note that data processing often cannot rectify poor-quality data. Therefore, not all collected data may be useful.
Ensuring high data quality involves also several key steps: loading, transforming, modeling, aggregating, and assembling data for business use. During data loading, it’s important to make sure the data is imported correctly without errors. Transforming data involves cleaning and reformatting it to fit business needs, while modeling ensures the data structure follows established rules and logic, including checks for missing or empty fields. Aggregating data combines it in a way that’s easy to analyze, and assembling it for business use involves ensuring it meets all necessary validation rules. Following these steps ensures the data is accurate, reliable, and ready for effective decision-making, but handling it all manually can be very time consuming or even impossible.
Utilizing the right platform for data management can help to ensure high-quality data and help save time and effort for the data team. A robust data management platform provides tools for data governance, enabling consistent application of data quality standards and facilitating real-time monitoring of data issues. These capabilities are vital for maintaining data integrity from initial entry to final analysis.
In the modern business environment, collecting and utilizing quality data is no longer just beneficial; it’s essential for operations and development.
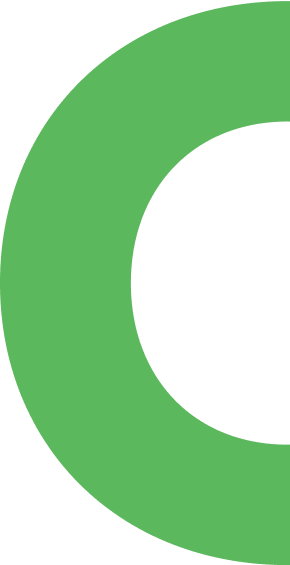
SelectZero free Starter tier
Over the years we have met with hundreds of members from different data teams to discuss their needs and use cases involving Data Observability. To this day, one of the most common ways engineers and [...]
Release 2025.5
Automated data validation suggestions based on profilings It is now easier than ever to create data tests automatically. By analyzing historical profiling data, the system can now suggest relevant validation rules based on [...]
Why is the importance of Data Quality growing
In today's rapidly evolving digital landscape, data underpins virtually every aspect of business operations, from strategic decision-making, to regulatory compliance and customer engagement. As the volumes and complexity continue to surge exponentially, compiling and maintaining [...]
Case study: SEB Baltics
Improving data quality and compliance Case study with SEB Baltics THE CUSTOMER SEB Baltics provides universal banking including advisory services to private individuals and all corporate customer segments in Estonia, Latvia and [...]
Release 2025.2
Starter edition of the platform A free version of SelectZero platform for data quality management is now available, making it easier than ever to improve data reliability. More information about the functionality is available here [...]
Case study: Citadele Bank
Ensuring data quality & regulatory compliance in banking Case study with Citadele THE CUSTOMER Citadele is a universal bank offering a wide range of banking products to retail, SME, and corporate customer [...]